Stable Kernel Sunspaces-based Anomaly Detection
The decentralization of the electric grid and the sophistication of recent cyberattacks require distributed detection methodologies that respect the power system physics. This fundamental observation motivated the development of an attack detector that is based on data-driven subspace methods. In more detail, the detector is constructed by identifying the system-under review's stable kernel representation (SKR), i.e., DG agent, in the attack-free case. Then local estimation models for each DG agent are developed to detect attacks in a distributed manner. The detectability of attacks is based on local data measurement aggregated at every agent, and any divergent operation from the SKR-defined model is perceived as a potential compromise.
The effectiveness of our physics-informed detection framework has been evaluated using the Canadian urban distribution feeder model and under three diverse attack scenarios, namely, attacks corrupting the measurement vectors, attacks targeting the control inputs, and load deviation attacks. In Figure 1, we present normalized residuals under a simulated control input attack. The outlined threshold (Δ_th) is used for the distributed detection of anomalous behavior that could compromise the operation of the DG agent.
Figure 2 demonstrates the residual dynamics during the real-time operation of the Canadian model in which DG #2 is under attack from 0.25s until 0.45s. Using our SKR-based detection, tight decision boundaries can be established, overcoming potential contingencies and promptly mitigating them(e.g., via islanding) before they can develop into system-wide threats.
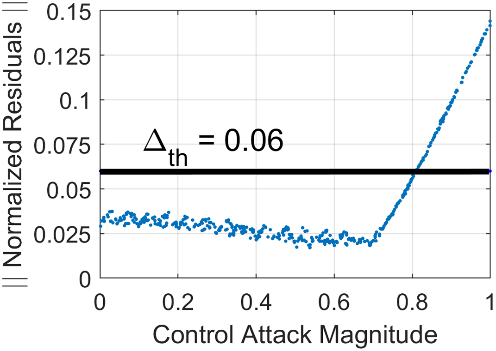
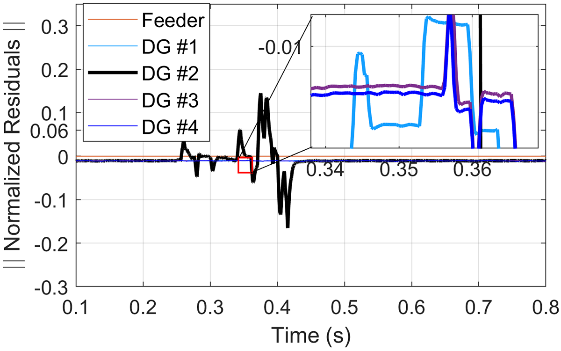
I. Zografopoulos and C. Konstantinou, "Detection of Malicious Attacks in Autonomous Cyber-Physical Inverter-Based Microgrids," in IEEE Transactions on Industrial Informatics, vol. 18, no. 9, pp. 5815-5826, Sept. 2022, doi: 10.1109/TII.2021.3132131.